Scaling Biology 002: Gevorg Grigoryan, Co-Founder and CTO at Generate:Biomedicines
On building a machine learning-powered generative biology platform with the ability to create new drugs on demand across a wide range of biologic modalities.
Welcome to Decoding Bio, a writing collective focused on the latest scientific advancements, news, and people building at the intersection of tech x bio.
Today we’re chatting with Gevorg Grigoryan, CTO and co-founder of Generate:Biomedicines. Grigoryan’s career started as a post-doc at the University of Pennsylvania. In 2011, he joined Dartmouth College where he remains an Associate Professor in computer science, biology and chemistry. In 2017, convinced by the potential of data-driven protein design, Grigoryan and the Flagship Pioneering team founded Generate:Biomedicines. Grigoryan is now CTO and oversees the company’s platform.
Generate:Biomedicines in a snapshot
Technology
The Generate Platform comprises an integrated loop of generative biology models with internal data generation, ingestion and measurement for continuous improvement. Generative biology enables the company to produce never-before-seen therapeutic proteins with unprecedented efficacy. The tightly integrated machine enables the company to rapidly advance their models and build a competitive moat in the form of proprietary data and expertly utilized infrastructure. Ultimately, the platform enables the company to design a range novel biologic therapeutics (complex antibodies, enzymes, and cytokines) against a range of difficult-to-drug targets.
One of the company’s key models is called Chroma, a diffusion generative model that creates new protein molecules based on geometric and functional programming instructions. The model was recently published here.
Chroma notably achieves (1) linear (over quadratic) scaling (2) likelihood evaluation (3) programmable generation of desired function using conditioner models.
Pipeline
Generate:Biomedicines has a broad pipeline spanning immunology, oncology and infectious disease. The team has successfully dosed their first patient with GB-0895 and GB-0669.
Partnerships
The company has three disclosed partnerships:
Amgen: $50M upfront to discover and create protein therapeutics for five clinical targets (Jan 2022). The partnership was expanded in Jan 2024 to include a sixth target.
Roswell Park: Strategic collaboration to discover and develop CAR-T-cell therapies for up to three targets (November 2023).
MD Anderson Cancer Centre: Strategic collaboration to jointly discover and co-develop protein therapeutics for up to five oncology targets in advanced cancers (April 2023).
Financing
In total, Generate:Biomedicines has raised nearly $700M in equity financing since 2020. Most recently, the company announced a $273M Series C in September 2023. This financing round attracted many new investors including Amgen; NVentures, ; MAPS Capital and Pictet Alternative Advisors. Earlier investors include Flagship Pioneering and Fidelity Management & Research Company.
Interview with Gevorg Grigoryan
Your career started in academia firstly as a post-doctoral student at the University of Pennsylvania and then as a professor at Dartmouth. What inspired you to start researching computational, data-driven approaches to understanding and desgining protein structure?
During my postdoctoral studies, I noticed many scientists could look at a protein structure and accurately reason about what it would and wouldn’t do, and whether a design would work or not. A lot of this came from experience, and to achieve this mastery I realized I’d either have to wait a few decades or find another approach. This led me to explore whether we could use data-driven and statistical methods to develop such mastery much faster.
I posed a thought exercise: if a new structure walks in the door, and you have seen nothing similar to it before, how would you design a sequence for it using data-driven methods?
Using statistical methods we were able to design from scratch sequences that could fold into such proteins. This was amazing to me as the perception had been that proteins were too complicated to be appropriately represented or parameterised in this way. I realized that there is something truly special going on in the field of generative protein design.
Overtime, I came to believe that such techniques would have a lot to say in how we design proteins, but the field needed scale in terms of data ingestion and model size to make these approaches truly generalize to all proteins and have an impact across biologic drug discovery.
How did this realization lead to the founding of Generate:Biomedicines?
My biggest surprise was starting Generate, I never imagined I would be leaving academia - having suffered through getting tenure and establishing myself in the field. Around 2016 I contacted Noubar who was then a guest professor of entrepreneurship at MIT to discuss generative protein design and to understand whether there was interest for this idea in the commercial realm. We brought the idea (a data-driven over physics-based approach to protein structure prediction) to the Flagship Pioneering team.
I initially thought the idea could form a SaaS model to serve biologic drug discovery at various pharmaceutical companies. But the Flagship team wanted to be more bold. They wanted to build a biotech machine that produces its own broad suite of biologic drugs.
I was inspired by the enthusiasm and eagerness from the Flagship and venture capital teams. In academia I had initially faced pushback with academics saying “this isn’t going to work”; “we already have Rosetta”; “why do you need this?”.
How would you describe Generate Biomedicine’s strategy? How has this changed over time?
The strategy has changed a lot since the company’s founding in 2018. At first, the goal was to demonstrate that these statistical protein-design concepts work and can build truly generalisable models. Five years later, the tools can generate such designs at unprecedented fidelity. So the question is no longer about “how do I do ‘x’ with a protein?”, but has shifted to “if I can design any function within a protein, how do I maximize patient impact?”
Our strategy is to build a company or machine that creates its own data, feeds off that data, becomes better and produces biologics to impact patient lives. We aim to integrate data-driven approaches further towards the clinic with these earlier discovery methods into one seamless end-end data-driven machine.
Please expand on Generate Biomedicine’s data-driven methods closer to the clinic?
The premise for our discovery engine is to run multiple programmes that have their individual priorities. As we collect data, this feeds back into a central model. As our two lead programmes enter the clinic, we want to extend this concept to clinical data. We want to build the data infrastructure, connectivity and informatics layer to integrate the clinical data we collect into a central model to help limit and streamline clinical testing into the future. Ideally as an industry we should be limiting the amount of human testing we do. So the question now becomes how can we extract more data and information from every patient we test?
Generate recently announced it had spent $30M on structural biology labs in Andover. Can you explain the importance of internal data generation to the company and how you think about what types of data to generate?
Structural data is important for producing generalisable models. Whilst the PDB offers good coverage, there are clear under-represented areas: particularly structures of protein-protein and antibody-antigen interactions. Given Generate’s mission of biologic discovery and development, we are focusing on generating these types of structures at scale. We also do not just focus on generating structures relevant to our targets of interest, but aim to be diverse in our generation. Given our aim to create a data generation and utilization machine, this investment is also contributing to solving structural biology bottlenecks to create a cutting-edge and efficient process.
The majority of protein models are good at predicting function in isolation, however the next step is for these models to accurately describe how proteins function in the context of neighbors and eventually in the context of larger cellular and sub-cellular structures. We believe our new Andover labs will enable us to tackle such questions.
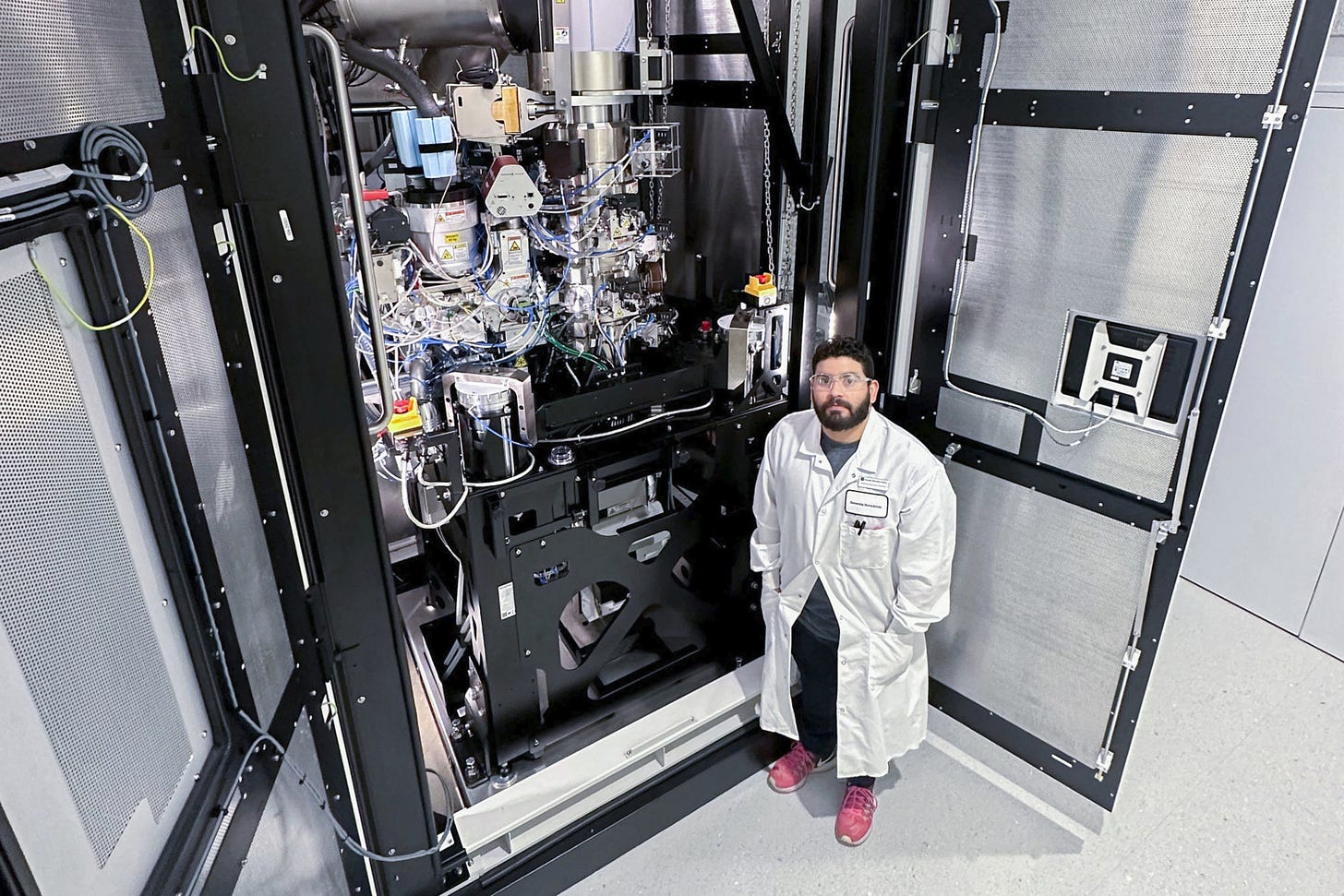
Aside from internal data generation and collection, do you focus on any external data acquisition?
We think about this all the time. On the clinical side, our two partnerships with MD Anderson and Roswell Park are in part motivated by the data they have in specific patient populations. On the molecular side, we have also considered this but the tricky part is that most of the data sources out there have not been collected or organized with machine learning models in mind. This creates an extra hurdle when attempting to use this to train our models.
Let's talk about Chroma, your generative model for protein design, what was the rationale for publishing the model, open-sourcing the weights and the key insights from the Generate team?
We were driven to publish the model because it seemed wrong to sit on such an advance that has so much potential, even outside of biology. We felt we had something interesting to say about protein design and wanted to give back to the community. The most important advances in the paper focused around scalability and the approach to conditioning. Achieving linear over quadratic scaling enables the efficient and rapid generation of large multi-chain protein structures. In terms of conditioning, as a proof of concept, we showed that the conditioning framework is flexible enough to condition on text, so one can describe the desired protein function in plain language and have the required protein generated.
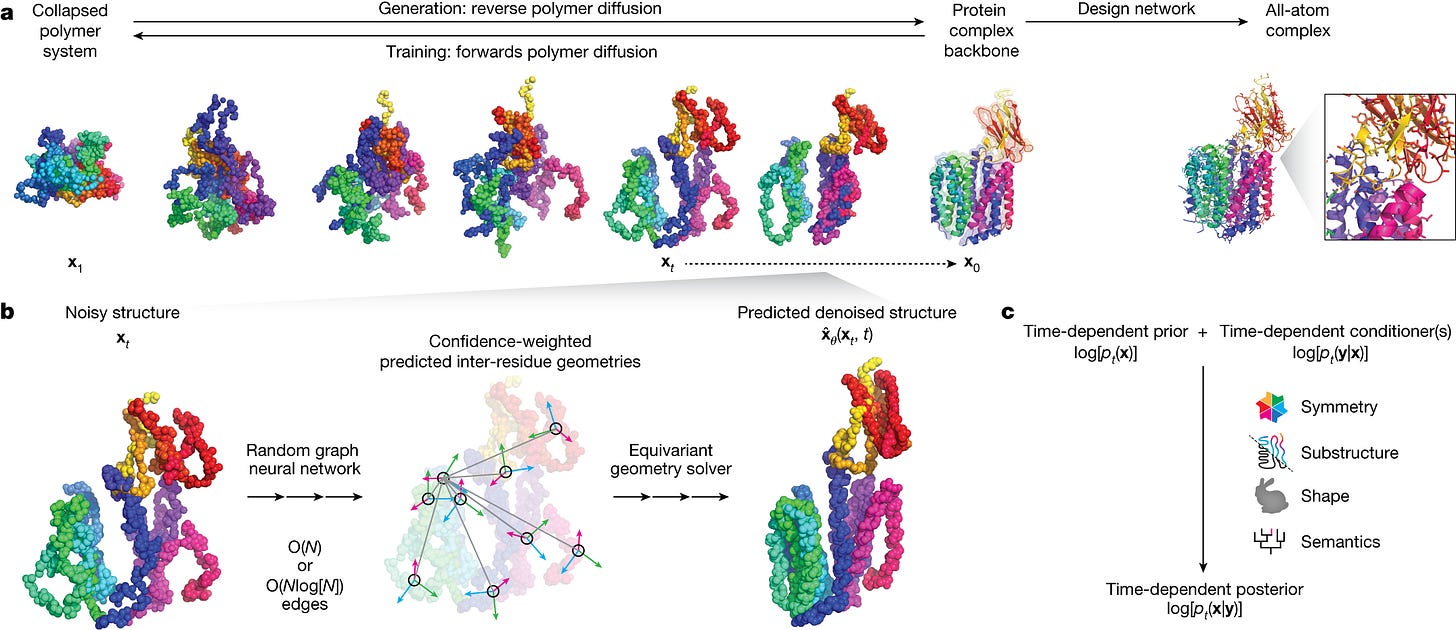
Since Chroma’s publication, how have you advanced the model or ways of thinking?
The most important advance in my eyes would be the approach to conditioning - being able to generate a protein with desired properties and function.
The question now is how do you further the models capability in conditional generation, how do you build good potent conditioners that represent different biological functions and properties? This side is more difficult as it requires the generation and ingestion of specific datasets according to the property you’d like to condition on. For example, to know if a protein structure will fold at a given pH or if an enzyme would catalyze a certain reaction would need information from specific assays).
How do you pick drug programmes to prosecute? Do the strengths and weaknesses of The Generate Platform play a role?
For our early programmes, we picked well-validated targets with minimal biological and commercial risk. We didn’t want to compound such risks with being an early company that hadn’t collectively taken a compound into the clinic.
Now, the programmes we choose are getting progressively more bold. We more frequently use our de novo generation platform to prosecute targets that may normally have not been considered.
On a more personal note, what is an informative experience that has shaped your view of the world?
I grew up in Armenia in the former Soviet Union and witnessed its collapse aged only 11. I have seen wars, economic struggle and a country in the depths of poverty. When I came to the USA I saw the contrast. A lot of how I judge character and events are with this experience in mind. I actually am thankful for that experience. Because, again, in times of crises, you see extremes. You see incredible kindness that you could never think would have happened, and just unbelievable cruelty and everything in between. You learn from that spectrum.
Do you have any unconventional advice for aspiring biotech founders?
Always be driven by a scientific problem, the solution is secondary. If you are motivated in this way, you will never be disappointed as the science is always beautiful.
NB: sentence structure changed in some cases to improve readability
Ggod wishes to the team at Generate. More and more we see in widely scattered fields the shift toward data-driven modeling. But we can't relinquish the older physics-based models either- best is to use them as co-partners, the two wings of the aircraft of research.